Machine Learning for Ad Targeting: Enhancing Precision and Efficiency in Ad Campaigns
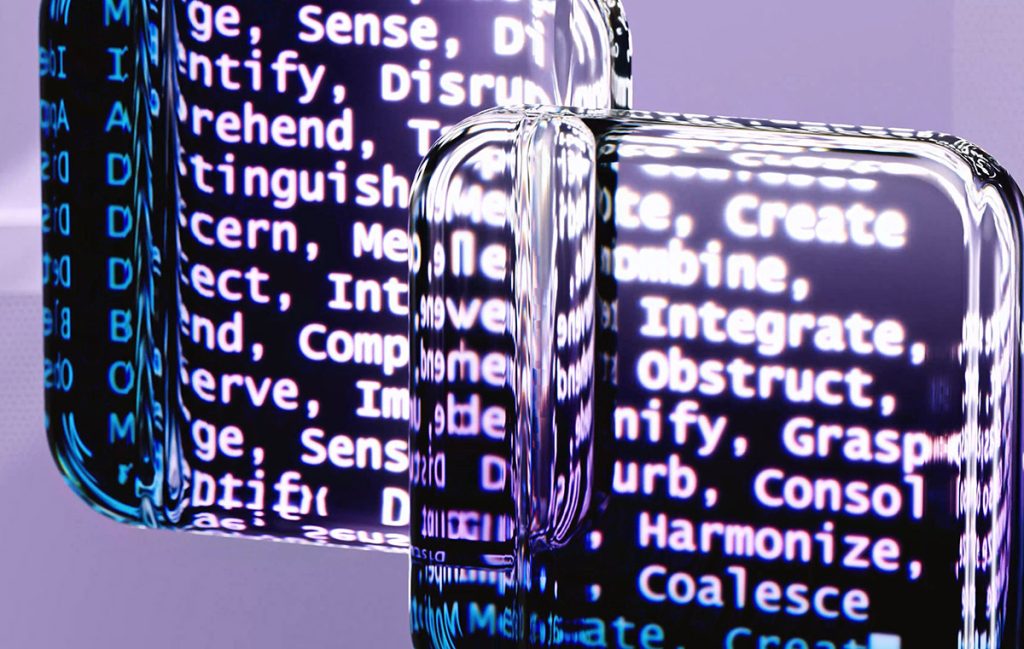
Machine learning has revolutionized the way businesses approach ad targeting, offering a solution that enhances precision and efficiency in ad campaigns. Traditional methods have often struggled with the challenges of reaching the right audience at the right time, leading to inefficiencies and wasted resources. In this post, we’ll delve into the importance of ad targeting, the limitations of traditional approaches, and how machine learning presents a game-changing solution. Understand in detail the pivotal role of machine learning in transforming the ad targeting landscape, providing businesses with the tools they need to navigate the fast-paced world of digital advertising.
Understanding Ad Targeting in Marketing
In today’s digital age, reaching the right audience has become pivotal in the realm of advertising. The process of ad targeting holds the key to amplifying conversion rates while minimizing wastage of ad spend. As marketers, understanding the challenges of traditional ad targeting methods and embracing innovative strategies can significantly enhance precision and efficiency in ad campaigns.
Challenges in Traditional Ad Targeting
Traditional ad targeting methods have long been plagued by inherent limitations that hinder their effectiveness in reaching the desired audience segments. The conventional demographic and behavioral targeting approaches, while valuable, are not without their drawbacks.
Explore the Limitations:
- Inaccuracies: Traditional methods often suffer from inaccuracies, leading to ads being displayed to an audience that may not resonate with the offering. This can result in wasted resources and missed opportunities to connect with potential customers.
- Lack of Real-time Adaptability: One of the major challenges is the lack of real-time adaptability. Static demographic data and historical behavioral patterns may not always reflect the current interests and needs of the target audience, leading to suboptimal ad placements.
- Inefficiency in Reaching Niche Audiences: Traditional ad targeting methods can struggle to effectively reach niche audience segments. This limitation hinders the ability to tailor ad content to specific, highly targeted groups, potentially diluting the impact of the advertising message.
By acknowledging these challenges, marketers can begin to appreciate the necessity of evolving their ad targeting strategies beyond traditional methods. Embracing the potential of machine learning in ad targeting can pave the way for dynamic, precise, and efficient campaigns that resonate with the right audience at the right time.
In the upcoming sections, we will delve deeper into the transformative impact of machine learning on ad targeting, exploring how it mitigates the limitations of traditional methods and empowers marketers to drive unparalleled results in their advertising endeavors.
Introduction to Machine Learning in Ad Targeting
In today’s digital age, the effectiveness of ad campaigns heavily relies on the precision of ad targeting. Machine learning has emerged as a game-changer in revolutionizing ad targeting strategies, empowering businesses to reach the right audience with the right message at the right time. By leveraging advanced algorithms and predictive analytics, machine learning has reshaped the advertising landscape, enabling marketers to delve deep into user behavior patterns and preferences. Let’s delve into this powerful technology and explore its applications in enhancing precision and efficiency in ad targeting.
Understanding Machine Learning Algorithms
At its core, machine learning involves the development of algorithms that can analyze data, identify patterns, and make decisions with minimal human intervention. In the context of ad targeting, these algorithms continuously analyze and learn from user interactions, enabling advertisers to tailor their campaigns based on real-time insights. By leveraging machine learning, marketers can unravel complex data sets and gain a comprehensive understanding of consumer behavior, leading to more effective targeting strategies.
Optimizing Ad Targeting Strategies
Machine learning algorithms are pivotal in optimizing ad targeting strategies by identifying relevant user segments and predicting their responses to different ad creatives. By leveraging historical data and real-time user interactions, these algorithms can identify the most responsive audience segments and personalize ad content to maximize engagement. This proactive approach allows advertisers to create seamless and personalized ad experiences, ultimately driving higher conversion rates and ROI.
Enhancing User Experience and Engagement
Beyond precise targeting, machine learning contributes to enhancing the overall user experience by delivering relevant and compelling ad content. By leveraging burstiness in user behavior, machine learning algorithms can adapt ad delivery based on dynamic user preferences and trends. This adaptive approach ensures that ads remain relevant and engaging, creating a more immersive and less intrusive advertising experience for users.
Conclusion
As we continue our exploration of machine learning in ad targeting, it becomes evident that this technology holds the key to unlocking unprecedented precision and efficiency in ad campaigns. By harnessing the power of advanced algorithms and data-driven insights, businesses can embark on a quest to connect with their target audience in a more meaningful and impactful way. In the following sections, we will delve deeper into specific use cases and best practices for implementing machine learning in ad targeting, providing actionable insights for marketers looking to stay ahead in today’s fast-paced digital landscape.
Data Collection and Processing for Ad Targeting
In today’s digital age, the foundation of effective ad targeting lies in the meticulous collection and processing of data. Understanding the intricacies of data collection and processing is crucial for marketers looking to enhance precision and efficiency in their ad campaigns.
Data Collection:
The process of data collection for ad targeting involves gathering information from various sources, including user interactions, browsing history, and demographic details. Each piece of data is like a puzzle piece that helps paint a clear picture of consumer behavior and preferences. User interactions, such as clicks, searches, and social media engagement, provide valuable insights into individual interests and intent. Browsing history offers a glimpse into the websites visited, products viewed, and content consumed, guiding ad targeting towards relevant offerings. Demographic information, including age, gender, location, and socio-economic status, adds another layer of depth to the data, allowing for tailored ad delivery based on specific audience segments.
Data Processing:
Once the data is collected, the next step is processing it to extract actionable insights for ad targeting. Data processing involves analyzing, categorizing, and interpreting the collected information to identify patterns, trends, and opportunities. Advanced algorithms and machine learning techniques play a pivotal role in processing large volumes of data with speed and accuracy. By delving into the data, marketers can unravel nuanced consumer behaviors, interests, and preferences, enabling them to create targeted ad campaigns that resonate with their audience.
In conclusion, the synergy between data collection and processing forms the backbone of precision in ad targeting. By leveraging the wealth of data available and employing robust processing techniques, marketers can unlock the potential for delivering highly relevant and effective ad campaigns that capture the attention of their target audience.
Algorithmic Ad Optimization
In the world of digital advertising, algorithmic ad optimization plays a pivotal role in enhancing the precision and efficiency of ad campaigns. Through the use of machine learning algorithms, advertisers can fine-tune their targeting strategies, ensuring that the right message reaches the right audience at the right time. Let’s delve into how predictive modeling, clustering, and personalized targeting based on user preferences and behavior are revolutionizing the ad optimization landscape.
Predictive Modeling: Anticipating User Behavior
Predictive modeling, powered by machine learning algorithms, enables advertisers to anticipate the behavior of potential customers. By analyzing historical data, such as past interactions and conversions, these models identify patterns and trends that can be used to predict future user actions. This proactive approach allows advertisers to tailor their ad content and delivery based on the likelihood of specific outcomes. By leveraging predictive modeling, advertisers can embark on a quest to reach their target audience with unparalleled precision, maximizing the impact of their ad campaigns.
Clustering: Segmenting Audiences for Tailored Messaging
Clustering algorithms are instrumental in segmenting audiences based on shared traits and behaviors. By categorizing users into distinct clusters, advertisers can unravel valuable insights about different audience segments. This segmentation facilitates the creation of personalized ad content that resonates with specific groups, leading to higher relevance and engagement. Just as a world-class chef carefully curates ingredients to create a seamless blend of flavors, clustering empowers advertisers to craft ad campaigns that deliver a tailored experience for each audience segment.
Personalized Targeting: Tailoring Ads to User Preferences
Personalized targeting, a core tenet of algorithmic ad optimization, involves tailoring ad content based on individual user preferences and behavior. Machine learning algorithms analyze user data to discern preferences, interests, and past interactions, allowing advertisers to deliver highly relevant and personalized ad experiences. By leveraging this approach, advertisers can cultivate a sense of connection with their audience, fostering a sense of loyalty and trust. In the fast-paced world of digital advertising, personalized targeting is a game-changer, enabling advertisers to leverage data-driven insights to create ad campaigns that resonate on a deeply personal level.
In conclusion, the utilization of machine learning algorithms for ad optimization heralds a new era of precision and efficiency in digital advertising. Through predictive modeling, clustering, and personalized targeting, advertisers can harness the power of data to craft ad campaigns that speak directly to the hearts and minds of their audience. As the world of digital advertising continues to evolve, the resilience and adaptability of algorithmic ad optimization stand as a testament to its pivotal role in shaping the landscape of modern marketing strategies.
Enhancing Precision and Efficiency with Machine Learning
Machine learning has revolutionized the field of ad targeting, offering unparalleled precision and efficiency in ad campaigns. By harnessing the power of advanced algorithms and predictive analytics, machine learning enables marketers to optimize ad targeting in ways that were previously unimaginable.
Predictive Analytics for Targeting
Machine learning algorithms analyze vast amounts of data to identify patterns and trends, allowing for highly accurate predictions of consumer behavior. By leveraging historical customer data, these algorithms can anticipate the preferences and purchasing habits of individual users, enabling precise ad targeting that aligns with their interests and needs.
Real-Time Optimization
One of the key advantages of machine learning in ad targeting is its ability to adapt and optimize in real-time. By continuously analyzing incoming data and user interactions, machine learning algorithms can make instantaneous adjustments to ad targeting strategies, ensuring that ads are delivered to the most relevant audiences at the optimal times.
Improved ROI and Customer Engagement
The enhanced precision and efficiency made possible by machine learning directly translate to improved ROI and better customer engagement. By serving ads to the right people at the right time, marketers can maximize the impact of their campaigns, driving higher conversion rates and ultimately increasing the return on their ad spend. Additionally, delivering personalized and relevant content to users fosters a deeper level of engagement, leading to stronger customer relationships and brand loyalty.
Advanced Segmentation and Personalization
Machine learning enables advanced segmentation and personalization, allowing marketers to tailor ad content to specific audience segments with unprecedented accuracy. By understanding the nuanced preferences of different user segments, advertisers can deliver highly targeted and personalized ad experiences that resonate with individual users, driving higher levels of engagement and conversion.
Conclusion
The transformative impact of machine learning on ad targeting is clear. Through predictive analytics, real-time optimization, and advanced personalization, machine learning has elevated the precision and efficiency of ad campaigns, ultimately leading to improved ROI and enhanced customer engagement. Marketers who embrace these capabilities are poised to thrive in an increasingly competitive digital advertising landscape.
Real-time Ad Personalization
In the constantly evolving landscape of digital advertising, real-time ad personalization has become a game-changer, primarily driven by the seamless integration of machine learning algorithms. This transformative technology enables advertisers to dynamically adjust ad content and targeting parameters in real-time, based on user interactions and evolving trends. Let’s delve into how machine learning is revolutionizing ad personalization, enhancing precision, and efficiency in ad campaigns.
Adaptive Content Tailoring
Machine learning algorithms analyze user behavior and preferences in real-time, allowing advertisers to tailor ad content dynamically. By leveraging these insights, ads can be personalized to resonate with individual users, creating a more compelling and relevant experience. This proactive approach to content customization not only increases user engagement but also contributes to a more seamless and thrilling user journey.
Dynamic Targeting Optimization
With the aid of machine learning, ad targeting parameters are continually optimized based on real-time data streams. By harnessing the power of algorithms to interpret user responses and interactions, advertisers can refine their targeting strategies to align with the evolving interests and preferences of their audience. This fast-paced adaptation ensures that ad campaigns remain resilient and effective, even in the face of rapidly changing market dynamics.
Enhanced User Experience
Real-time ad personalization not only benefits advertisers but also greatly enhances the overall user experience. By presenting users with ads that are closely aligned with their interests and intent, machine learning creates a more personalized and tailored online environment. This personalized approach significantly reduces ad fatigue and ensures that users are presented with meaningful and contextually relevant content throughout their online journey.
Predictive Decision-Making
Machine learning models use historical and real-time data to make predictive decisions on ad personalization, enabling advertisers to anticipate user preferences and behavior. This predictive capability empowers advertisers to embark on a quest for greater effectiveness and efficiency in their ad campaigns, aligning their strategies with user interests and market trends. By leveraging the power of predictive analytics, advertisers can unravel powerful insights to inform their ad personalization strategies.
Conclusion
Real-time ad personalization powered by machine learning is undoubtedly a pivotal advancement in the realm of digital advertising. It not only offers advertisers the ability to create more engaging and targeted campaigns but also ensures that users are presented with ads that seamlessly integrate with their online experience. As machine learning continues to shape the advertising landscape, the dynamic nature of ad personalization through real-time adjustments will undoubtedly remain a critical component in achieving advertising success.
Behavioral Segmentation and Audience Profiling
In today’s digital age, understanding consumer behavior is crucial for creating effective advertising strategies. With machine learning, precise segmentation of audiences based on behavior, interests, and intent becomes achievable, allowing for targeted messaging and personalized ad experiences.
Understanding Behavior Patterns
Machine learning algorithms analyze vast sets of data to identify patterns in user behavior. By examining online interactions, such as clicks, searches, and social media engagements, it becomes possible to categorize individuals based on their preferences and habits. For example, someone who frequently searches for fitness-related content and follows wellness influencers on social media may be classified as health-conscious.
Tailoring Ads for Specific Interests
Once behavior patterns are recognized, advertisers can tailor their ad content to resonate with specific audience segments. By leveraging machine learning insights, businesses can craft messaging that aligns with the interests and preferences of their target demographics. For instance, an athletic brand can create personalized ad experiences for the health-conscious segment by showcasing fitness apparel and workout accessories.
Predicting Purchase Intent
Furthermore, machine learning facilitates the prediction of purchase intent by identifying signals indicative of an individual’s readiness to make a purchase. By analyzing factors such as browsing history, cart activity, and time spent on product pages, advertisers can gauge the likelihood of conversion. This enables them to strategically deliver ads at opportune moments, increasing the chances of driving favorable outcomes.
Delivering Personalized Experiences
Audience profiling through machine learning empowers advertisers to deliver personalized experiences that resonate with individual consumers. By understanding the nuances of each segment’s behaviors and preferences, brands can create ad campaigns that feel bespoke and relevant, fostering stronger connections with their target audience. This personalized approach enhances engagement and cultivates a sense of rapport between the brand and the consumer.
In essence, behavioral segmentation and audience profiling, powered by machine learning, revolutionize ad targeting by ensuring that the right message reaches the right people at the right time. As businesses harness the capabilities of this technology, they can elevate the precision and efficiency of their ad campaigns, ultimately driving higher engagement and conversions.
Optimizing Ad Spend and Conversion Rates
In the realm of digital advertising, optimizing ad spend and conversion rates is crucial to the success of ad campaigns. With the utilization of machine learning, advertisers can significantly enhance the precision and efficiency of their ad campaigns by identifying high-value audience segments and predicting conversion probabilities. Let’s delve into how machine learning plays a pivotal role in achieving cost-effective and impactful ad campaigns.
Identifying High-Value Audience Segments
Machine learning algorithms analyze large sets of data to identify patterns and trends that may be imperceptible to human analysts. By doing so, they can pinpoint the specific audience segments that are most likely to engage with a particular ad. These high-value segments could be based on demographic information, past browsing behavior, or purchasing history. This level of precision ensures that ad spend is directed towards the most promising leads, maximizing the return on investment.
Predicting Conversion Probabilities
One of the remarkable capabilities of machine learning is its proficiency in predicting conversion probabilities. By leveraging historical data and real-time interactions, machine learning models can forecast the likelihood of a user converting after interacting with an ad. This predictive power allows advertisers to allocate their budget towards the most promising opportunities, thereby increasing conversion rates while minimizing wasteful expenditure.
Cost-Effective Ad Campaigns
With the insights provided by machine learning, advertisers can optimize their ad spend to ensure that every dollar is utilized most effectively. By directing resources towards the audience segments with the highest potential for conversion, ad campaigns become more cost-effective and yield a higher return on investment. This level of precision would be nearly unattainable without the analytical capabilities of machine learning algorithms.
In conclusion, machine learning has transformed the landscape of ad targeting by enabling advertisers to optimize ad spend and conversion rates through the identification of high-value audience segments and the prediction of conversion probabilities. As the digital advertising landscape continues to evolve, leveraging machine learning becomes an essential quest for advertisers looking to stay ahead in the fast-paced and competitive world of ad campaigns.
Challenges and Considerations in Implementing Machine Learning for Ad Targeting
Implementing machine learning for ad targeting presents various challenges and considerations that require thoughtful analysis and proactive measures for addressing potential issues.
Ethical Implications and Bias in Ad Targeting Algorithms
Adopting machine learning for ad targeting raises ethical considerations regarding algorithmic biases and discrimination. These algorithms have the potential to perpetuate biases based on factors such as race, gender, or socioeconomic status. It’s crucial for businesses to implement ethical oversight and regularly evaluate their algorithms to mitigate such biases. Ensuring diverse representation within the teams building these algorithms can also aid in identifying and addressing potential biases.
Data Privacy and User Consent
The significance of data privacy regulations cannot be overstated in the context of machine learning for ad targeting. As businesses leverage user data for personalizing ad experiences, obtaining explicit consent becomes paramount, aligning with regulations such as GDPR and CCPA. Balancing personalization with privacy involves transparent communication with users regarding data collection practices and providing easily accessible options for opting out of targeted advertising. By prioritizing user consent and data privacy, businesses can build trust and credibility while delivering tailored ad experiences.
By acknowledging and addressing these ethical and privacy challenges, businesses can harness the potential of machine learning for ad targeting while demonstrating a commitment to transparency, fairness, and user-centric practices.
The Future of Ad Targeting: Advancements in Machine Learning
As technology continues to evolve, the future of ad targeting is poised for remarkable advancements driven by machine learning. Let’s delve into the exciting developments and trends that are set to shape the landscape of ad targeting in the coming years.
AI-Driven Ad Creative Optimization
In the future, machine learning algorithms will play a pivotal role in optimizing ad creatives, ensuring they resonate seamlessly with the target audience. These algorithms will leverage big data to unravel patterns and trends, enabling marketers to craft compelling and personalized ad content that truly captivates the audience’s attention. The fast-paced evolution of AI-driven ad creative optimization is set to redefine the way brands engage with their customers, delivering a truly immersive and tailored advertising experience.
Cross-Device Targeting
With the proliferation of digital devices, cross-device targeting will be a game-changer in the ad targeting landscape. Machine learning will enable marketers to leverage user data across various devices, creating a seamless and cohesive ad journey for the consumer. By embarking on a quest to understand consumer behavior across different devices, advertisers can unlock new opportunities for engaging with their target audience at every touchpoint, driving enhanced brand visibility and engagement.
Hyper-Personalization
Hyper-personalization is set to be a pivotal force in the future of ad targeting, empowered by machine learning capabilities. Through the unrivaled leveraging of data insights, advertisers will be able to craft personalized ad experiences that resonate with individual consumers on a profound level. This level of personalization will unravel a new world of advertising, where every interaction feels tailored and meaningful, fostering stronger consumer-brand relationships and driving increased conversion rates.
In the fast-paced and ever-evolving digital advertising landscape, these advancements in machine learning for ad targeting are poised to be transformative, reshaping the way brands connect with their audiences and unlocking new dimensions of efficiency and precision.
Takeaways
In conclusion, machine learning offers significant benefits for enhancing ad targeting precision and efficiency in ad campaigns. By leveraging advanced algorithms and data analysis, marketers can optimize ad delivery to reach the right audience at the right time, ultimately improving ROI and customer engagement. However, it’s crucial to emphasize the importance of responsible and ethical implementation of machine learning in ad campaigns. Marketers should prioritize transparency, consent, and data privacy to build trust with consumers and uphold ethical standards in advertising practices.
When utilized effectively, machine learning can revolutionize ad targeting strategies, enabling advertisers to deliver more personalized and relevant content to their target audience. With a focus on responsible implementation, the integration of machine learning in ad campaigns can drive positive outcomes for both businesses and consumers, shaping the future of digital advertising in a transparent and ethical manner.